This article is only available in Dutch 🇳🇱
Many companies are getting on the AI train with no clear direction, assuming that simply implementing AI will solve their problems and - in some cases - help them achieve the latest standards for organizational innovation. And that's not surprising. A huge amount of fuss has surrounded AI in recent years, fueled by the success of generative models, chatbots and automation tools. AI is revolutionizing businesses and transforming industries. More than ever, we hear people saying a resounding "yes" when asked if they are using AI for work or personal matters. If your idea of an AI strategy does not go beyond chatbots, then you are missing the bigger picture, and this blog is for you.
From hype to strategy: shaping a meaningful AI approach
AI is much more than automating simple tasks. It has the potential to reshape the way you do business and interact with customers. Having a good idea of what you want to do with AI for your business is not enough to achieve the success you want. You need a good strategy. Between the AI skeptics who are fed up with the hype and the AI maximalists who believe that literally everything is solvable with AI, there are also agencies like us, with a more pragmatic approach. We believe that a good AI strategy is not only about empowering people and improving customer experiences, but also about realizing efficiencies that lead directly to more results-driven operations.
AI not only supports people but also improves processes, allowing you to achieve concrete results faster, more efficiently and with a clearer focus. A successful AI strategy starts at the core of your business. AI is not a magic bullet, but a powerful tool that, when deployed strategically, can help achieve organizational goals by both increasing efficiency and optimizing performance. A good AI strategy starts at the core of the business. That may sound very logical, but remember this: AI is not a magic bullet that will help you achieve your organizational goals. You need to think of it as a tool.
Wondering how to get past the hype and develop a strategy that really works for your business? In this blog, you'll find five steps for creating a good and meaningful AI strategy that goes beyond superficial solutions.
Step 1: Align AI with business goals
The first step is to understand that AI is a means and not an end in itself. A good AI strategy is closely aligned with your company's goals. First, determine what goal you want to achieve: do you want to improve the customer experience, optimize internal processes, or drive innovation in product development? Once you've identified the key areas that fit your strategic goals, you can explore how AI can help achieve them. For example, if you want to reduce customer turnover, machine learning models can predict the likelihood of turnover and recommend retention strategies. However, the combination of human expertise and AI remains crucial to creating the most effective solutions. In improving operational efficiency, AI-based process automation can take over repetitive tasks, allowing employees to focus on more strategic and valuable work. Customization ensures that AI solutions best fit your organization's unique needs and challenges.
Some of our partners aim to reduce the large number of customer service calls about parking reservations. Despite having advance reservation options available through the website or app, customers still often call with questions about booking details, changes or cancellations. How can AI help with that?
In this case, an AI-powered virtual assistant could be integrated into the website and app to handle common questions about parking, but with a focus on personalized assistance. Instead of giving general answers, the assistant should be able to make statements about specific reservations made by logged-in users in the appropriate context. For example, the assistant could provide real-time answers to questions about confirming booking details, provide step-by-step guidance on changes and process cancellations automatically, all tailored to the individual customer. In addition, a machine learning model could predict peak times when customers are likely to call, allowing the system to proactively send reminders, confirmations or updates to the customer's phone or email. This AI-powered solution would streamline the process, reduce customer frustrations and free up customer service agents to handle more complex inquiries. The result is not only improved operational efficiency, but also a better and personalized customer experience.
Step 2: Understand the full spectrum of AI capabilities
AI is not just about automating repetitive tasks. Many companies mistakenly believe that AI is solely about automation and replacing human labor. While automation is valuable, it is only one part of the whole. Consider how AI can complement or enhance human decision-making, rather than seeing AI as a way to remove humans from processes. For example, AI systems in healthcare can quickly analyze medical images, allowing doctors to focus on diagnosis and treatment. In this scenario, AI processes the repetitive, data-intensive tasks, while humans add context and critical thinking skills. This collaborative approach, in which AI works alongside humans rather than replacing them, is crucial to ensure long-term success.
Achieving sustainable results with AI requires integration that improves efficiency and decision-making, without neglecting the human aspect in areas such as creativity, empathy or complex problem solving. AI is actually good at making judgments, provided it is based on models that are bias-free as much as possible. In that case, AI can provide a much broader perspective on things by objectively analyzing large amounts of data and discovering patterns that may remain invisible to humans.
However, if companies rely solely on AI without human collaboration, they risk losing valuable human insights, experiencing resistance from employees and frustrating customers due to a lack of personalized service. While AI can provide powerful insights, human interpretation remains essential, especially in situations that require nuance and empathy. The combination of AI and human judgment is therefore crucial.
Step 3: Involve domain experts
AI solutions should not be left to IT departments alone. Although it is a technical discipline, the most valuable applications come from those who know the business inside out: your domain experts. These are the people closest to your customers, processes and products. They can best identify the gaps where AI can add the most value, but also play a crucial role in the success of implementation.
However, there is a risk that these experts may resist AI because they may fear that parts of their expertise and activities will be taken over by the technology. Therefore, it is critical to not only involve them in the development of AI solutions, but also give them ownership in the end result. By making them co-owners of the AI solution and making them understand that AI is not a threat, but rather a tool that can enhance their work, you can turn that resistance into commitment.This requires a transparent dialogue in which you explain that AI does not replace them, but supports them in their tasks, in which you focus on how it can contribute to more efficiency and value in their work.
By bringing domain experts into the AI story, they not only feel heard, but also responsible for the ultimate success of the implementation. This approach increases the likelihood of acceptance and adoption of the technology within the organization, and ensures that AI solutions are used to their full potential.
Step 4: Optimize AI through iteration and transparency
AI is based on probabilities, which means that it works based on patterns and predictions, and thus always has some degree of uncertainty. This is not a flaw, but a feature of AI that must be embraced. No model will be 100% accurate, and some AI projects will require adjustments before delivering real value. Yet in many cases, 95% accuracy is more than sufficient. Consider, for example, AI models that perform routine tasks such as predicting customer behavior or automating simple administrative processes. In such cases, a margin of error is acceptable because the cost of an incorrect prediction is relatively low.
However, what is a bigger concern than just aforementioned accuracy is the lack of traceability in AI. AI can come up with an answer, but it is often unclear exactly how that answer was arrived at. Especially when AI is used to provide advice, such as when recommending a product choice, it is difficult to understand why AI made certain decisions. For example, the AI may recommend that Package A of insurance X is best for a customer, but no one knows exactly why. This can lead to distrust in the outcome, especially when the decision is impactful.
Therefore, building trust in AI within the organization is essential. It is important not only to measure the results of AI, but also to analyze erroneous outcomes and use them to improve the models. A good AI strategy should focus on continuous improvement: every erroneous outcome provides an opportunity to retrain the model and make it more accurate. This creates a feedback loop in which AI gets better and better, while the organization learns to better interpret and rely on AI decisions.In short, it is not just about embracing the probabilistic nature of AI, but more importantly about creating transparency and trust in the outcomes so that AI can make a valuable and reliable contribution to organizational decision-making.
Step 5: Start small and scale up gradually
One of the most challenging aspects of embracing AI is not only the technology, but more importantly, its acceptance within the organization. While AI technology itself can be developed and applied quickly, it is the organization itself that is often the biggest obstacle. This is because implementing AI means not only adding new tools, but also modifying business processes, building trust and changing the way people work.
Why is this so? Because AI works on the basis of probabilities and predictive models, which always involves some degree of uncertainty. This requires a culture that accepts this uncertainty and understands that AI is a support tool, not a magic solution that is always error-free.
Building trust in AI takes time. Employees must learn to trust AI's outcomes, even when the decision-making seems complex and is not always fully traceable. This can be challenging, especially when AI makes decisions previously made by humans. Herein often lies the fear that AI will make human expertise obsolete, which can create resistance. To overcome this resistance, organizations must make it clear that AI is meant to support employees, not replace them. The process must be transparent, with AI decisions explainable and measurable, so that employees feel they have a grip on the results.
In addition, the organization itself must be given time to adapt to AI-driven processes. Business processes must be revised to take full advantage of the technology, and this takes time. If it were purely about technology, implementation could be quick, but technology is not the bottleneck here. It is the organization's acceptance of this technology that determines the speed of adoption.
Therefore, it is crucial to start small and scale incrementally. Focus initially on areas where AI can make the biggest impact and where quick successes can be achieved. This helps build trust, both among employees and decision-makers, and lays a solid foundation for further rollout. As the organization grows in its confidence and understanding of AI, more advanced applications can be implemented.
Conclusion
Building a good and meaningful AI strategy is not magic. AI is a tool that, when applied strategically, can help companies achieve real, measurable results. By aligning AI with your business goals, understanding the full scope of its capabilities, engaging domain experts and embracing its iterative nature, you can move beyond the hype and create a meaningful AI strategy that delivers long-term value for your organization.
Key insights for organizations
- What business problems can AI solve for us? Identify business challenges.
- How can we use AI to enhance human capabilities rather than replace them? Think beyond automation and explore how AI can collaborate with employees.
- Are we ready to iterate and engage the right people? Recognize that AI projects may require adjustments and that it is important to create a culture where learning and iteration are central to long-term success.
Want to know even more? Talitha will be happy to tell you more. You can find her details at the bottom of this page.
Sign up
Leave your details below and be the first to receive the Dutch Mobile App Trends Report in your inbox.
Beyond the latest trends
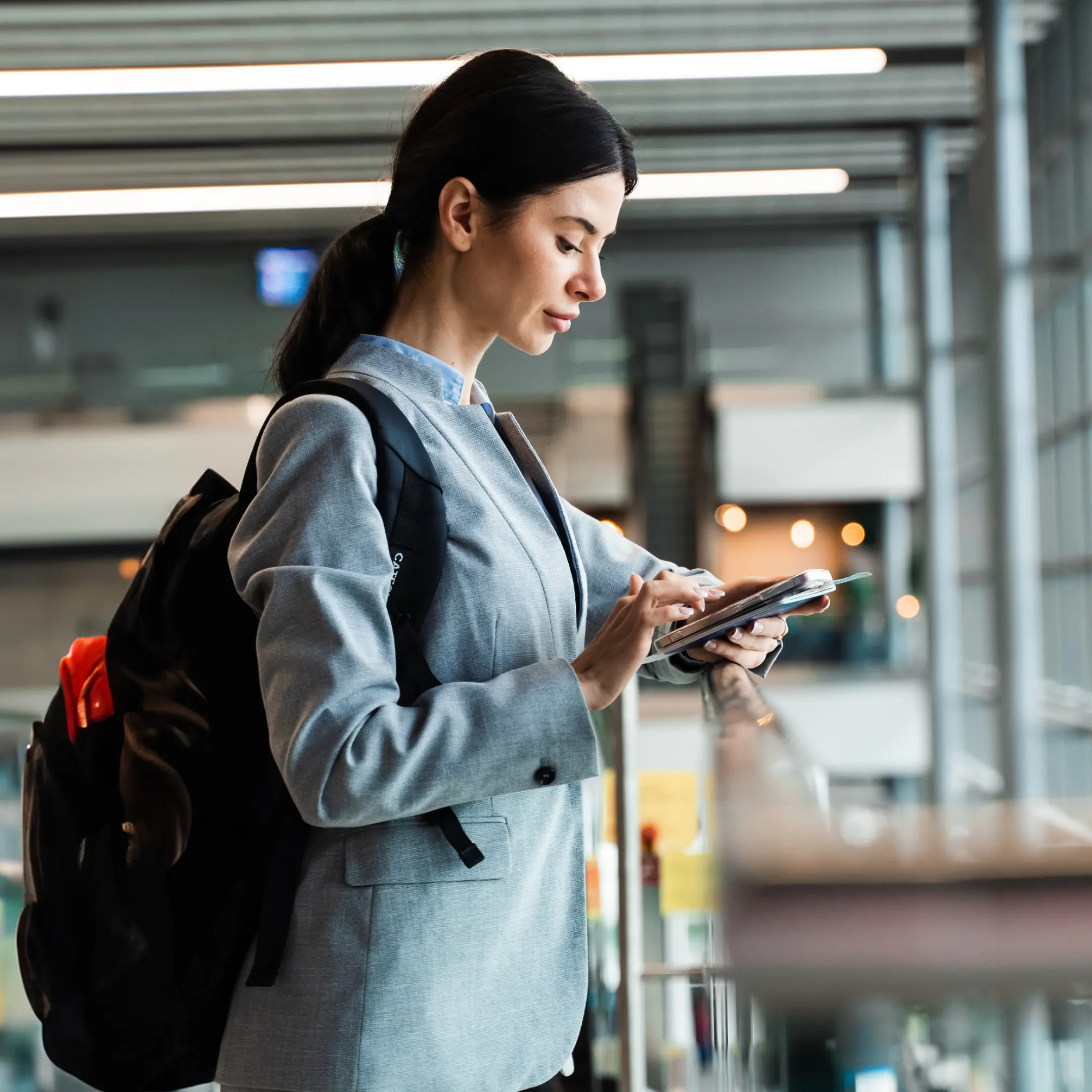
ADA Compliance: Digital Accessibility in Aviation
At Move, we have years of experience in developing digital passenger journeys. Read more about our approach around the ADA, WCAG and how to make sure you develop accessible digital solutions as a U.S. airport.
ADA Compliance: Digital Accessibility in Aviation
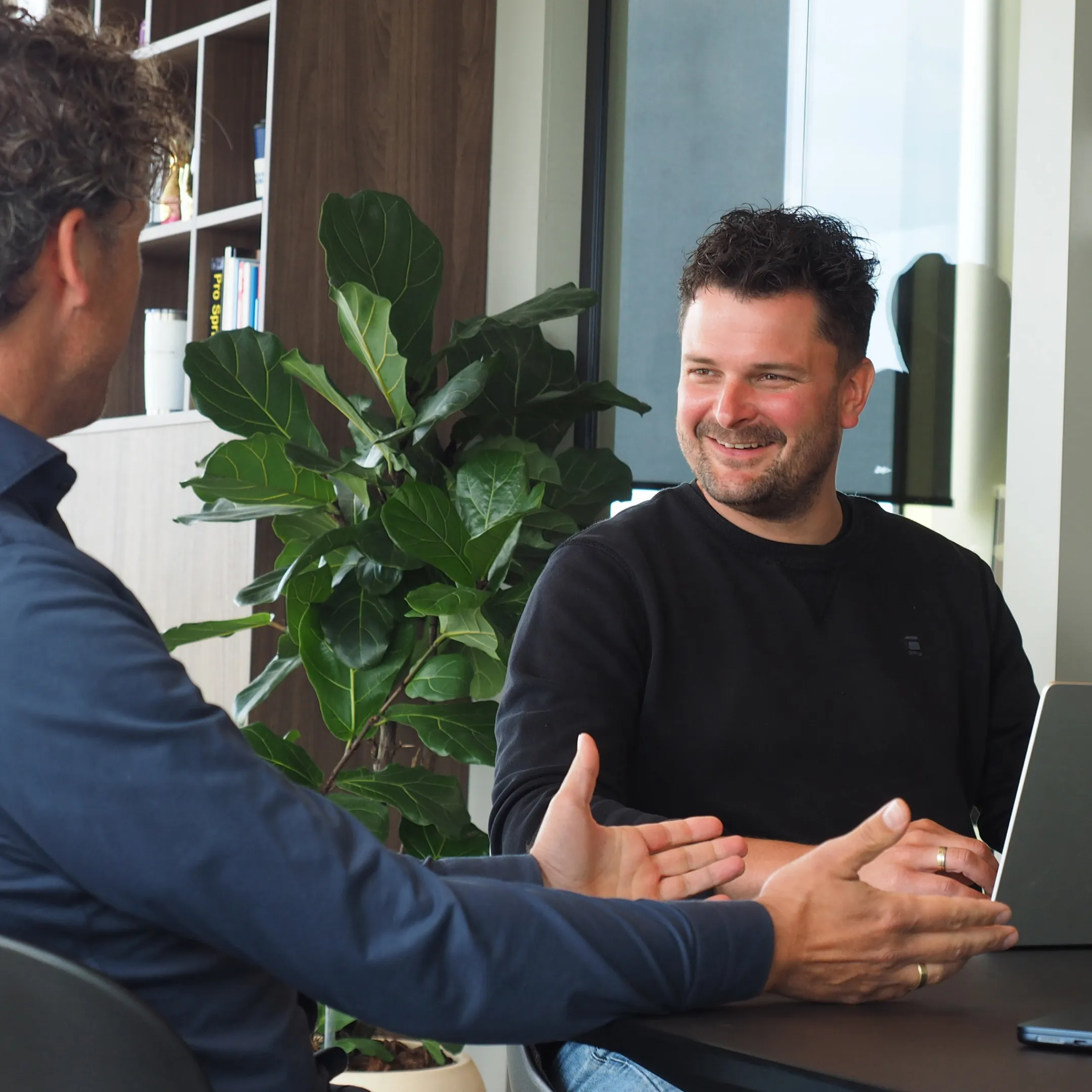
Cross-platform at Move
Our CTO Jan Gerard Gerrits on the use of cross-platform technology.
Cross-platform at Move
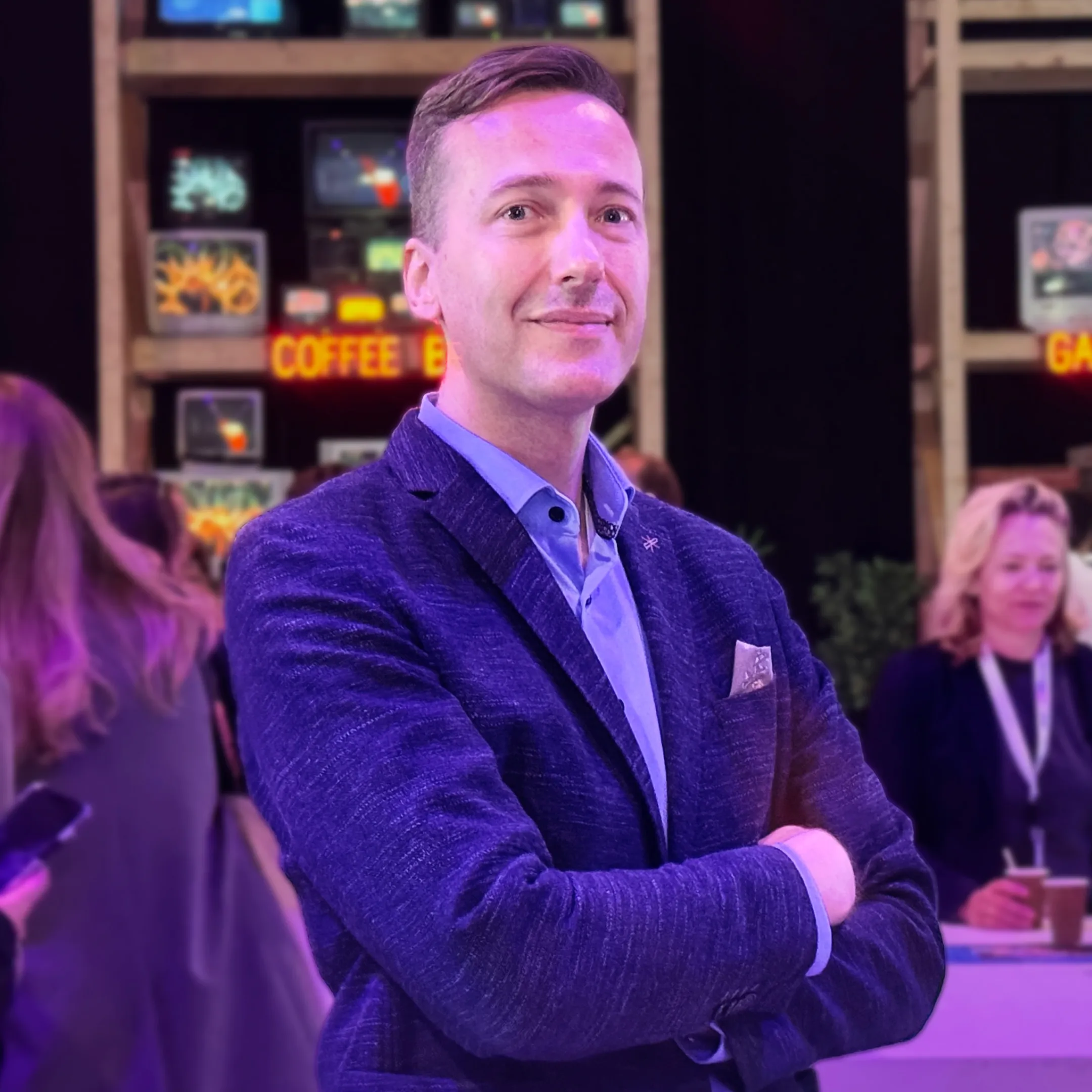
Partou x EDAY: Leon Koppenol's key takeaways
Leon Koppenol is Partou's Chief Digital Information Officer (CDIO). During Emerce EDAY '24, he took the stage to talk about Partou's digital transformation and strategic partnership with Move. In this article, read the key takeaways from his talk.